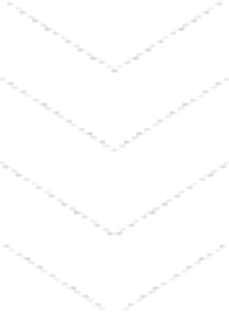
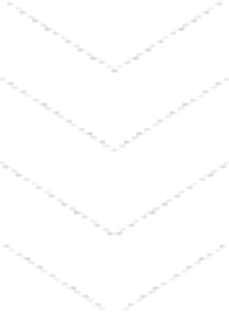
Protect Lite 2020
The new Protect Lite 2020 prevents the execution of malware and ransomware on all end points.
By leveraging machine learning and compacted computer hacking methods, our solution manages to predict malicious code quasi real-time pre-execution, without relying on signatures, heuristic rule sets or any other protection solutions.
Our new solution uses proprietary Dynamic Deception and Active Engagement Technology in order to detect in real-time and fully protect against infiltration of data destructive programs that utilise ZERO-DAY EXPLOITS as well.
What makes Protect Lite 2020 a revolutionary solution is its approach to ZERO DAY ATTACKS.
Suppose an intruder has managed to get a foot-hold on a network out of which he hopes to gain persistent access, data exfiltration, data corruption or system corruption as a whole.
If the attacker chains procedures to interact with the kernel at one point in order to lure the system or not, it becomes irrelevant because we hunt the final point. If they manage to get there, they get stopped or they get thrown in our deception sandbox.
We protect against these attacks by using machine learning algorithms and we also send alerts to users, which then serve as feedback loops. Data exfiltration is addressed purely with alerts on which we build our machine learning model for the system to keep learning continuously.
We started building Protect Lite 2020 by quantifying the best attack methods a hacker can leverage against a network to get to know the specific conditions that have to be met in order for the attack to be successful. In this way, we would be able to create a product capable of controlling the land, while misguiding the attacker.
In computer hacking, once you land on the endpoint, you have to live off the land. You utilize whatever legitimate means you have to escalate your privileges and ultimately pivot unto the network. Whether this is done by a human or by software, the playground is all the same.
Therefore, understanding the playground and controlling it is the first step in achieving control.
“We provide tracking-engagement mechanisms which entice a malicious software or human to take our course of action. The method is built on a mathematical model of a Cantor diagonalization argument and can be proved mathematically to be sound regardless of the attacker’s methods or payloads.
In this way, no ransomware or any other threat can affect the data.”
Sergiu Popa
CEO, Cyneward
Protect Lite 2020 focuses on advanced data management and threat intel so that you can say farewell to ransomware and hackers.
Advanced threat detection.
Ransomware, Malware (per total classes) and Network Compromise Detection
What we call Sieve 1 are mathematical models of Machine Learning which do static analysis of portable executables.
The sum of the parts is bigger than the whole approach.
Dynamic deception and ML combined with fractal approaches in Mathematics. Multiple models of detection.
Sieve 2 refers to mathematical models of detection for file less ransomware and “in-memory” threats.
Playground + Rules = Game.
We control the playground and the rules, so threats fall directly in our hands.
Boost your Sieves, fine tune them and let them work together.